WHITE PAPER SERIES
DATA MIGRATION
'A Business Guide to Data Migration' White Paper Series
In this 4 part white paper series, we summarise the lessons we have learnt from 20+years of data migration experience and expertise working with asset intensive industries. The white papers are designed to provide insights to executives of asset intensive organisations who are leading large-scale digital transformation or projects requiring complete data migration.
The 22 page white paper series includes:
1. Data Profiling & Remediation:
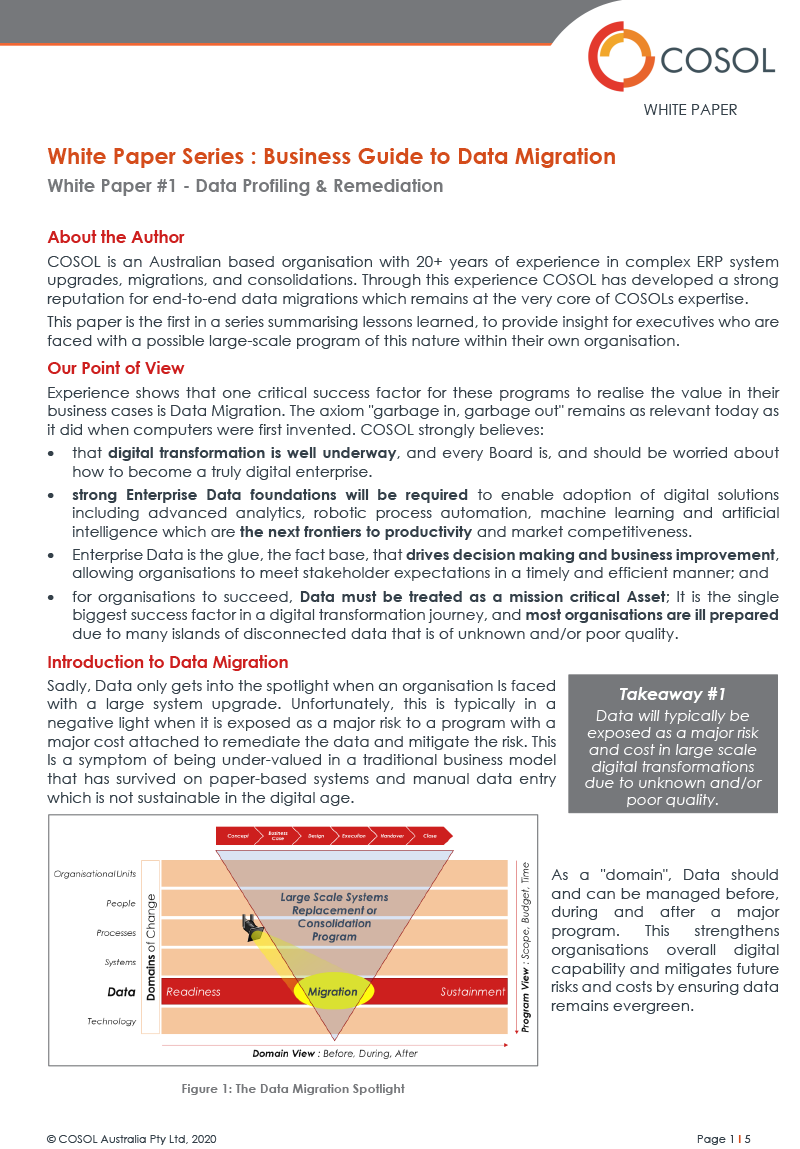
2. Data Standardising & Loading
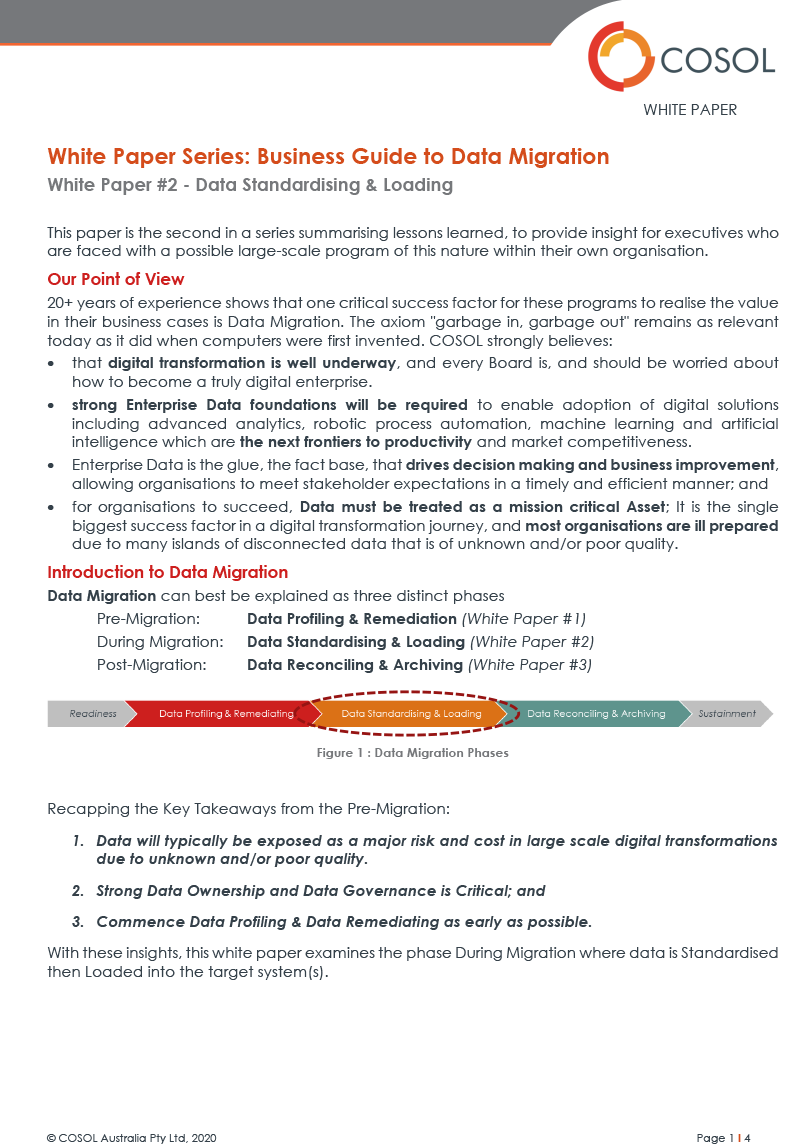
3. Data Reconciling & Archiving
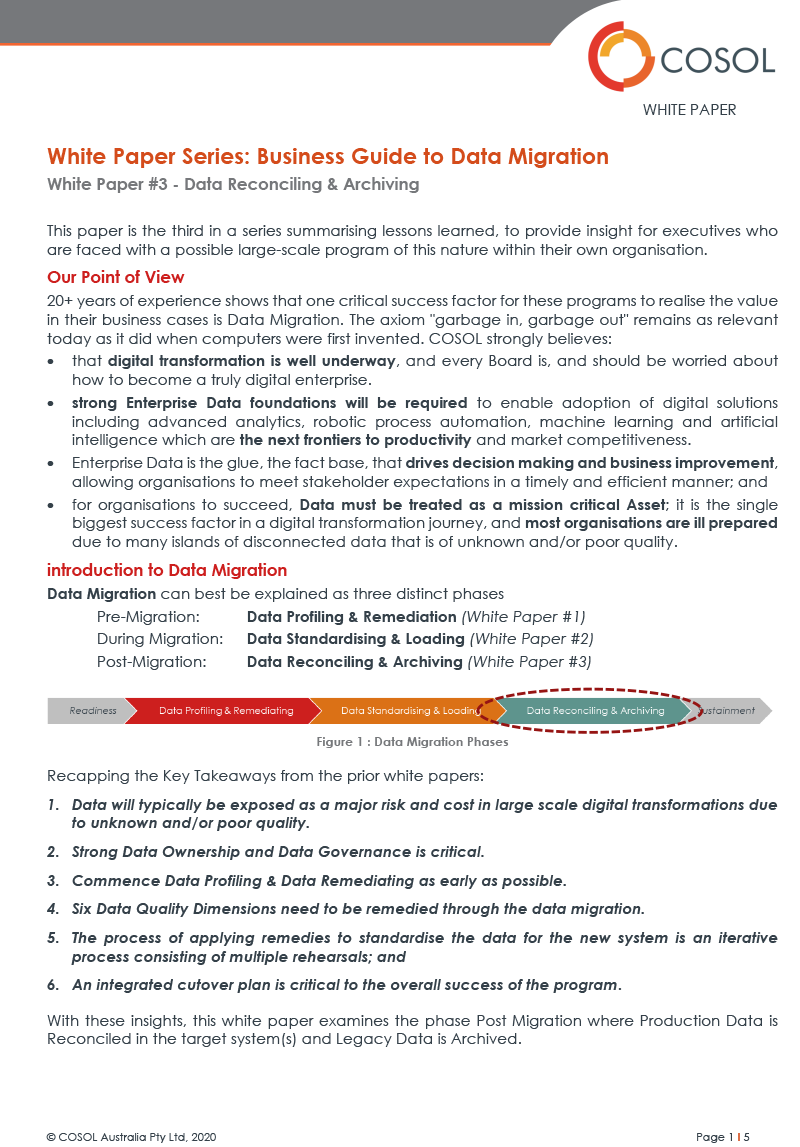
4. Practical Application (Best Practice)
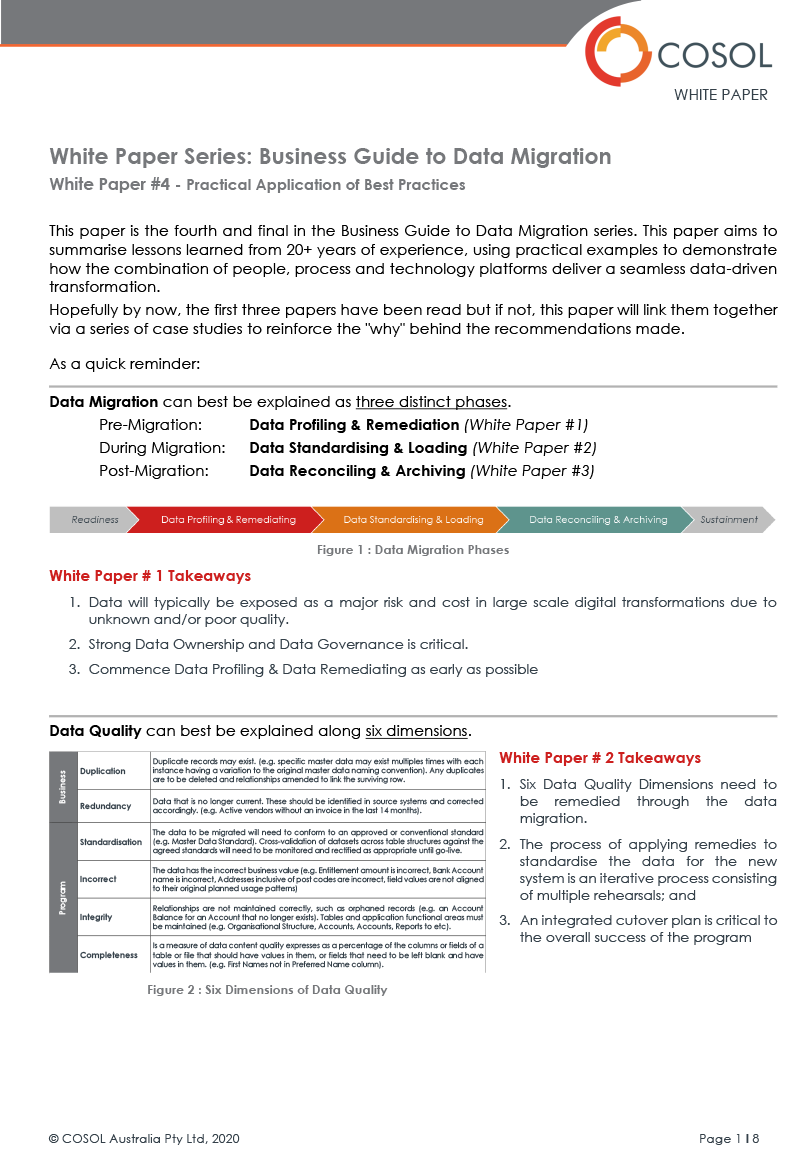
Complete form for instant access to all white papers:
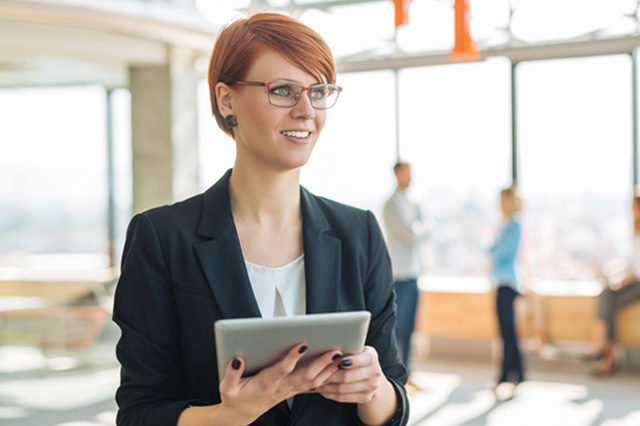